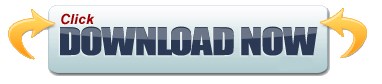
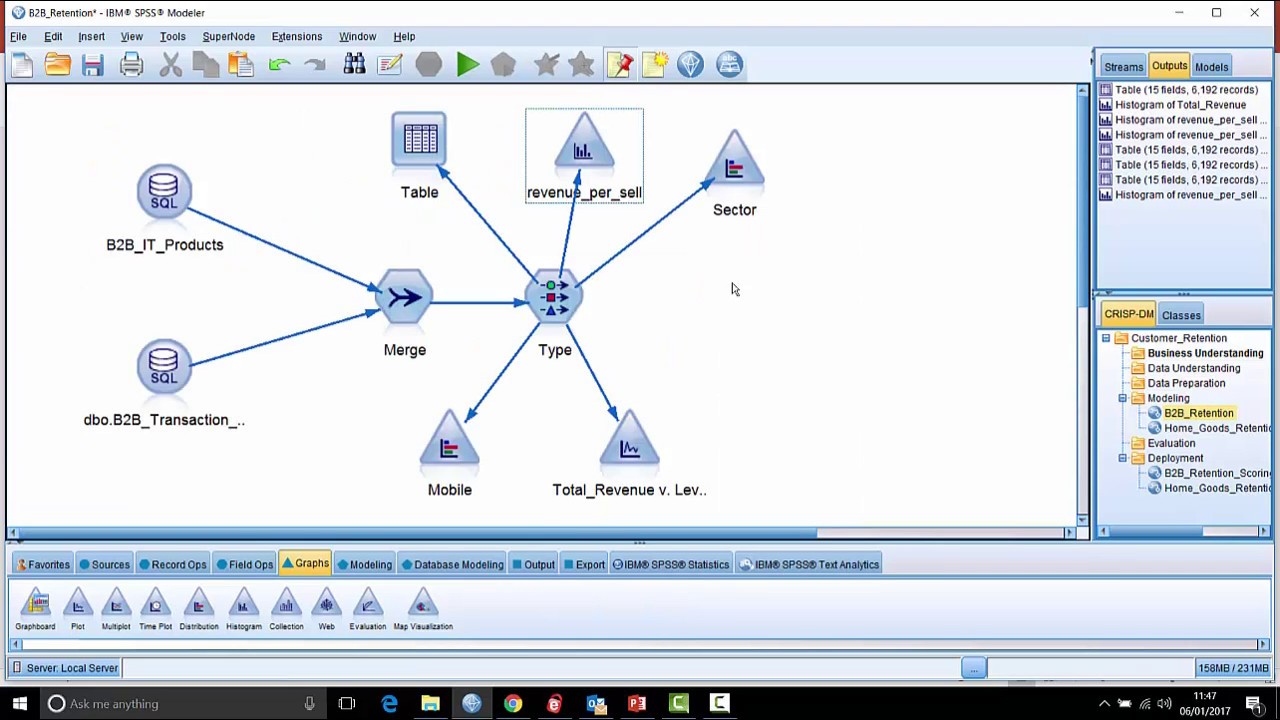

#Spss modeler 18 r how to#
The tutorial is divided into two parts: In the first part, you will understand the idea behind a Kernel method in Machine Learning while in the second part, you will see how to train a kernel classifier with Tensorflow. In statistics, kernel density estimation (KDE) is a non-parametric way to estimate the probability density function (PDF) of a random variable. Kernel density estimation Another common method of evaluating densities in multiple dimensions is kernel density estimation (KDE). Three algorithms are implemented through the same API: NaiveKDE, TreeKDE and FFTKDE.

Likelihood ratio at a point is ratio of class-1 likelihood estimate to class-0 likelihood estimate, times the class odds, where this is calculated as the posterior mean estimate under Beta(1, 1) prior, given the observations. The third figure compares kernel density estimates for a distribution of 100 samples in 1 dimension. distributions with occasionally large deviations from the mean (such as the distribution of portfolio losses). gaussian_kde to allow for heterogeneous sampling weights and thought the results might be useful for others. kalepy: a Python package for kernel density estimation, sampling and plotting. stats we can find a class to estimate and use a gaussian kernel density estimator, scipy. gaussian_kde * standard deviation of the sample. This post will show you how to: Use Matplotlib to represent the PDF with labelled contour lines around density plots.Kernel density estimation is a fundamental data smoothing problem where inferences about the population are made, based on a finite data sample. Gaussian kernel density estimation python stats module. Gaussian kernel density estimation python
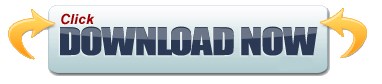